CAD2RL
Real Single-Image Flight Without a Single Real Image
Fereshteh Sadeghi Sergey Levine
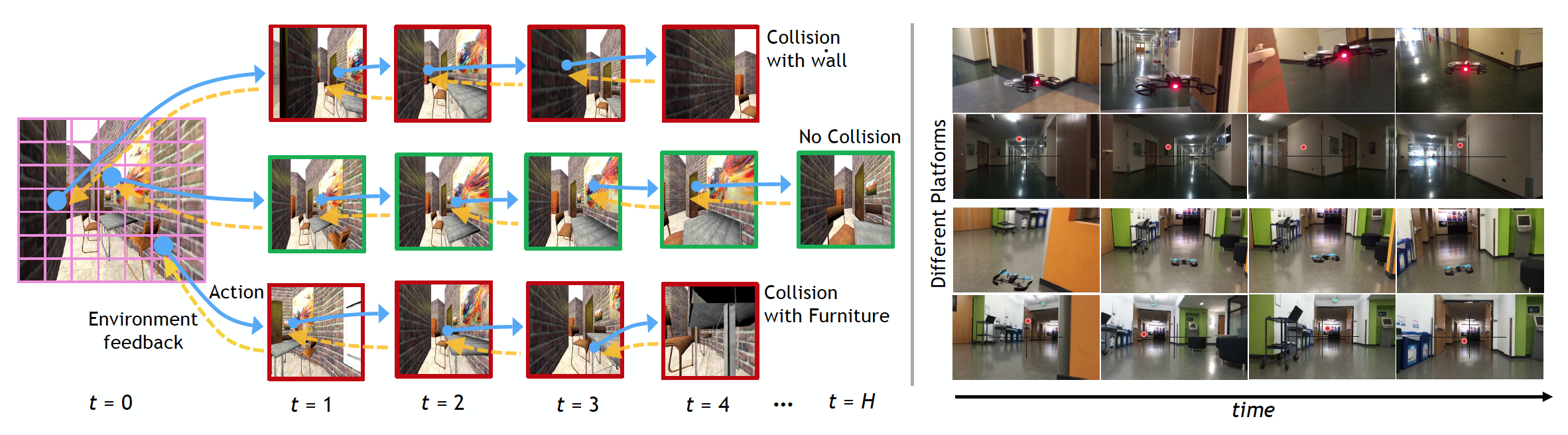
Abstract
Deep reinforcement learning has emerged as a promising and powerful technique for automatically acquiring control policies that can process raw sensory inputs, such as images, and perform complex behaviors. However, extending deep RL to real-world robotic tasks has proven challenging, particularly in safety-critical domains such as autonomous flight, where a trial-and-error learning process is often impractical. In this paper, we explore the following question: can we train vision-based navigation policies entirely in simulation, and then transfer them into the real world to achieve real-world flight without a single real training image? We propose a learning method that we call CAD$^2$RL, which can be used to perform collision-free indoor flight in the real world while being trained entirely on 3D CAD models. Our method uses single RGB images from a monocular camera, without needing to explicitly reconstruct the 3D geometry of the environment or perform explicit motion planning. Our learned collision avoidance policy is represented by a deep convolutional neural network that directly processes raw monocular images and outputs velocity commands. This policy is trained entirely on simulated images, with a Monte Carlo policy evaluation algorithm that directly optimizes the network's ability to produce collision-free flight. By highly randomizing the rendering settings for our simulated training set, we show that we can train a policy that generalizes to the real world, without requiring the simulator to be particularly realistic or high-fidelity. We evaluate our method by flying a real quadrotor through indoor environments, and further evaluate the design choices in our simulator through a series of ablation studies on depth prediction. For supplementary video see:
Bibtex
@inproceedings{sadeghi2017cadrl, title={{CAD2RL}: Real Single-Image Flight without a Single Real Image}, author={Sadeghi, Fereshteh and Levine, Sergey}, booktitle={Robotics: Science and Systems(RSS)}, year={2017} }